Sourced (DevGlobal) : How to ensure good quality labels for training data working with remote teams
“There’s power in mapping. Maps mean recognition – an acknowledgment that communities exist and have needs. To be on the map is to be counted for support and input and partnership and investment. Open and accessible maps are mission-critical for governments and humanitarians to get much-needed relief and development assistance to every community in need.”
The ramp project is working with the World Health Organization to map healthcare buildings, a tool that will support humanitarian emergency response teams in the likelihood of the next pandemic.
Radiant Earth Foundation, a nonprofit empowering organizations with open geospatial training data, models, and metadata standards, has been supporting the ramp project’s labeling efforts for building rooftops. Producing building footprint data is a methodical task that requires polygons to be labeled over satellite and drone imagery, usually by hand on a computer, to provide context or confirm the details of each polygon. The labels paired with the imagery become the training data inputs for a building footprint extraction model.
In this blog post, we discuss the labeling process, answering a fundamental question: How can we ensure generating high-quality labels working with remote teams?
TaQadam and B.O.T – Platform for geospatial annotation and socially responsible annotators
One way to produce training data labels is by annotating satellite and drone imagery. This task involves identifying a particular feature in the image that a person observes – e.g., a road or building – and tagging it accordingly. The model uses these labels to determine patterns and make predictions. Therefore, the more precise the label, the better the quality of training data that, in turn, produces more accurate predictions.
Radiant partnered with TaQadam and B.O.T. (Bridge. Outsource. Transform), who completed the annotation tasks. TaQadam is a geospatial annotation platform specializing in social impact projects that advance Earth observation data in building footprint, flood detection, damage assessment, crisis recovery, and agriculture value chain mapping. B.O.T. is the first impact sourcing platform in the Middle East and North Africa that creates income-generation opportunities for low-income communities. They provide services such as data collection and labeling, which freelancers from underprivileged communities accomplish, and an in-house expert team manages.
We started working with TaQadam in 2019 when they provided expert labeling for LandCoverNet, our global land cover classification training dataset based on Sentinel-2 satellite data. Our existing partnership with them and their extensive geospatial understanding meant an easier orientation for the ramp project. TaQadam has worked with various satellite imagery sources, including SPOT, Pleiades, Worldview, NAIP, and Planetscope. B.O.T., on the other hand, launched its pilot program in 2021 with UNICEF support, training 200 young women to become expert data annotators. In partnership with TaQadam, B.O.T. was able to deliver 20+ data-related projects.
TaQadam and B.O.T. assembled 50 of their best annotators in five teams, each working full-time on the ramp project, their most significant and challenging data labeling assignment to date. B.O.T. screened individual labelers for accuracy and consistency, and either provided additional training or released them if the task was too complex.
How to ensure high-quality labels
One of the principles for generating high-quality labels is labeling different regions, which will produce geographically representative training data that practitioners can use to train their models for better accuracy. To ensure high-quality labels, the labeling pipeline consisted of annotators drawing building footprints on the imagery and validators verifying the labels. This two-step process is critical for consistency and accuracy. People adding labels to features in the imagery also required training on guidelines and a constant feedback loop to review labeling efforts and adopt changes as needed.
Using this workflow, we first selected the areas of interest (AOIs) based on the heterogeneity and geographical diversity of the available high-resolution satellite imagery. We decided on eleven countries: Bangladesh, Philippines, Oman, Haiti, Sierra Leone, India, Malawi, St. Vincent, South Sudan, Myanmar, and Ghana to cover diverse building constructions in the countries that range from dense areas with small buildings, to informal settlements, and courtyards.
The next step was defining the criteria for a good label. The DevGlobal team developed detailed data labeling specifications outlining the label format for rooftop extraction for various complex scenes. Radiant’s team conducted online training with labelers, highlighting how best to annotate polygon labels for capturing structures. Despite the guidelines, some labelers remained unclear about what building structures to include in dense urban areas like Dhaka in Bangladesh, Manila in the Philippines, and Muscat in Oman.
The dense urban areas in Dhaka, Muscat, and Manila made it hard for labelers to distinguish between individual buildings leading to inconsistent digitization of building rooftops. Labels were either overlapping or incomplete, and shadows and fields were sometimes incorrectly labeled as buildings. This is a very complex problem, and in many cases, it’s hard to distinguish buildings even from high-resolution satellite imagery. An updated labeling guide was created for the second round of training, which helped annotators spot common errors and discern one rooftop from another, which in turn improved the accuracy of labels and made them consistent.
In addition to Dhaka and Muscat, labeling tasks for Haiti, Sierra Leone, and India are completed, while Malawi, St. Vincent, South Sudan, Myanmar, and Ghana are currently being labeled. Additional AOIs will be selected and annotated over the summer with a target goal of 97,500 unique chips that will be curated during a final quality assessment phase for inclusion in their models.
Using STAC to make the labeled data queryable and accessible.
The satellite data and accompanying labels used to compile the training data are stored with metadata compliant with the SpatioTemporal Asset Catalog (STAC) specification. STAC is an open metadata standard offering a uniform index to describe Earth data. It allows users to discover spatial data across time and cloud storage locations. We added STAC catalogs to each batch of labeled data to make sure it is easily queryable and accessible by the DevGlobal team – and when the time is ready for open access publication, we will use the catalog and ingest it to Radiant MLHub, the repository for open-access geospatial training data and models.
In addition to building footprints, Radiant MLHub STAC-compliant training datasets also include imagery with labels for crop and land cover types, clouds, marine debris, and floods.
Sourced from: https://dev.global/
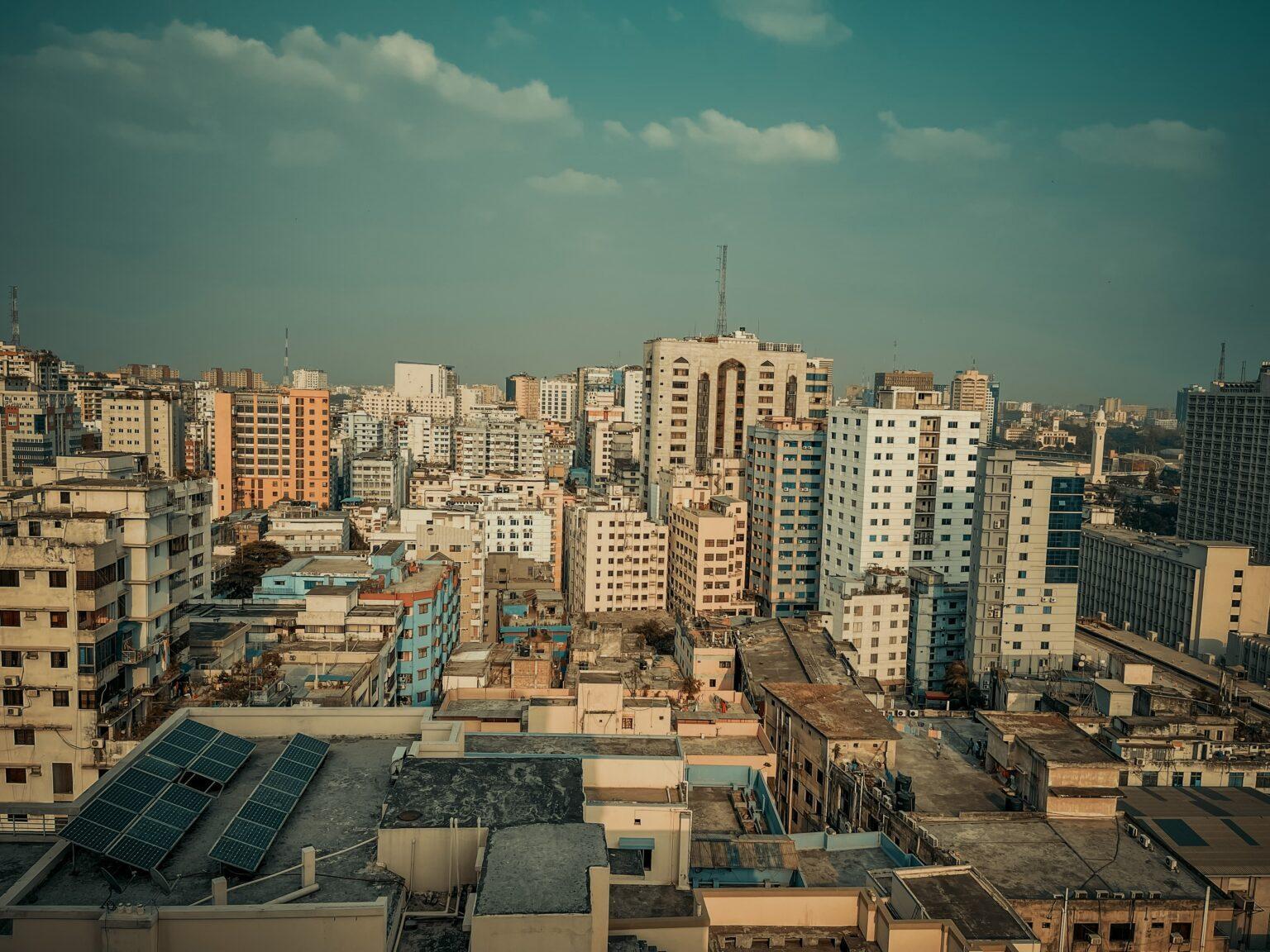