Sourced (Geospatialworld): Radiant Earth Foundation Releases Training Dataset for Global Land Cover Classification
The release completes the global dataset that guarantees highly accurate and scalable classification models across diverse geographies. The first version, released in 2019, contained image chips across Africa based solely on Sentinel-2 data. It was initially funded by Schmidt Futures, and NASA ACCESS and Microsoft Planetary Computer programs offered support leading to the completion of LandCoverNet, while Sinergise provided in-kind technology support.
“LandCoverNet is an essential benchmark for data scientists across the globe who wish to build advanced monitoring tools of our environment”, says Dr. Hamed Alemohammad, Executive Director and Chief Data Scientist, at Radiant Earth Foundation.
“It was born out of a community effort to improve the accuracy of global land cover maps. The applications built from this dataset will enable fully-automated and dynamic land cover classification algorithms using open-access satellite imagery,” he adds.
Cropland expansion, urbanization, and deforestation rates are among the changes that land cover maps can measure, providing critical insights into human and non-human activities that profoundly impact the global landscape. Scientists and policymakers can use the insights from these maps to help communities and governments meet Sustainable Development Goals (SDGs).
Real-world applications of land cover maps that measure our planet’s health include Impact Observatory’s automated annual global map and Google and the World Resources Institute’s recently released Dynamic World. While high-resolution satellite-based land cover maps are becoming more readily available, there is an overwhelming lack of open-access training datasets that will allow create thematic maps to monitor our natural resources on a global or regional scale or validate the accuracy of existing maps.
LandCoverNet identifies seven land cover class types: water, natural bare ground, artificial bare ground, woody vegetation, cultivated vegetation, (semi) natural vegetation, and permanent snow/ice. Each labeled pixel is also associated with a consensus score indicating the uncertainty from the human annotation process. These scores can help the model better learn the differences and similarities of each land cover class.
Radiant Earth has generated the training datasets from 300 geographically diverse tiles of ESA’s Sentinel-2 mission covering Africa, Asia, Australia and Oceania, Europe, North America, and South America. A total of 8,941 image chips of 256 x 256 pixels were labeled globally, resulting in ~586 million pixels for the entire training dataset.
What is training data?
Training data is the building block for producing Machine Learning models. In the case of land cover mapping, it contains satellite images along with labels specifying land cover classes present in the image. Models learn the pattern of these classes from the training data and can generate maps at large spatial scales. LandCoverNet supplies just that — training data for annual land cover classification that allows practitioners to build planetary change detection models on every continent of this world inhabited by humans.
“The availability and reusability of large-scale training datasets and models have increased the possibilities of advanced science applications of AI,” says Dr. Manil Maskey, Senior Research Scientist at NASA.
“As we move towards adopting open science principles within the NASA Science Mission Directorate, we envision engaging a broad community of experts to solve some of the challenging scientific problems using AI coupled with large-scale training datasets and benchmark models. Radiant Earth’s work in providing the opportunity to engage a broad community and infrastructure to support training datasets and models is instrumental in advancing AI for science with open science principles,” he adds.
“We are incredibly excited to see what people build using LandCoverNet. Training data is one of the biggest technical bottlenecks of progress with AI. Thus, this project provides incredible value to everyone, and doing so through Radiant Earth ensures that it finds its way to the hands of stakeholders that need it the most. At the end of the day, it is not what AI can do, but how it helps people and the planet”, says Dr. Bruno Sánchez-Andrade Nuño, Director, at Microsoft Planetary Computer.
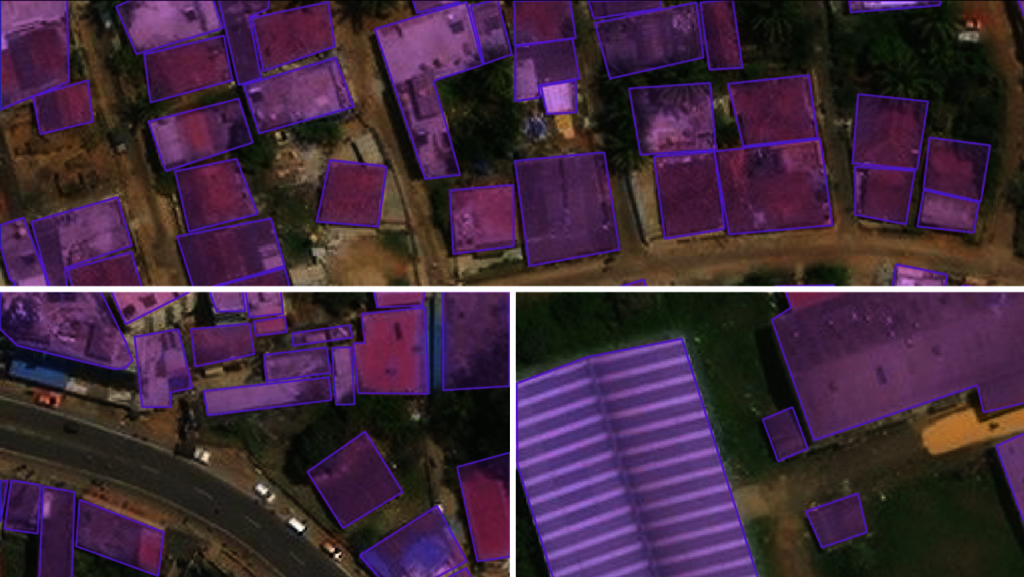